Road Freight in EU
Contents
10. Road Freight in EU#
We will pull data from eurostats and clean up the road freight data in EU.
10.1. Install Packages#
import pandas as pd
import numpy as np
import missingno as msno
import matplotlib.pyplot as plt
from matplotlib.colors import LogNorm
import seaborn as sns; sns.set()
import networkx as nx
10.2. Load Data#
Goods loaded in reporting country:
https://ec.europa.eu/eurostat/estat-navtree-portlet-prod/BulkDownloadListing?file=data/road_go_ia7lgtt.tsv.gz
Good unloaded in reporting country:
https://ec.europa.eu/eurostat/estat-navtree-portlet-prod/BulkDownloadListing?file=data/road_go_iq_utt.tsv.gz
source_loaded = "assets/road-freight-eurostats/road_go_ia_ltt.tsv"
source_unloaded = "assets/road-freight-eurostats/road_go_ia_utt.tsv"
df_load = pd.read_csv(source_loaded, sep='[\t|,]', na_values=[': ', ':'])
df_unload = pd.read_csv(source_unloaded, sep='[\t|,]', na_values=[': ', ':'])
/Users/leima/anaconda3/envs/theflow-code/lib/python3.7/site-packages/ipykernel_launcher.py:1: ParserWarning: Falling back to the 'python' engine because the 'c' engine does not support regex separators (separators > 1 char and different from '\s+' are interpreted as regex); you can avoid this warning by specifying engine='python'.
"""Entry point for launching an IPython kernel.
/Users/leima/anaconda3/envs/theflow-code/lib/python3.7/site-packages/ipykernel_launcher.py:2: ParserWarning: Falling back to the 'python' engine because the 'c' engine does not support regex separators (separators > 1 char and different from '\s+' are interpreted as regex); you can avoid this warning by specifying engine='python'.
df_load.sample(10)
unit | carriage | c_unload | geo\time | 2019 | 2018 | 2017 | 2016 | 2015 | 2014 | ... | 1991 | 1990 | 1989 | 1988 | 1987 | 1986 | 1985 | 1984 | 1983 | 1982 | |
---|---|---|---|---|---|---|---|---|---|---|---|---|---|---|---|---|---|---|---|---|---|
536 | MIO_TKM | HIRE | EU27_2007 | NO | 909.0 | 1147.0 | 1478.0 | 1306.0 | 1317.0 | 1251.0 | ... | NaN | NaN | NaN | NaN | NaN | NaN | NaN | NaN | NaN | NaN |
295 | MIO_TKM | HIRE | DK | CY | 2.0 | 2.0 | 2.0 | 1.0 | 0.0 | NaN | ... | NaN | NaN | NaN | NaN | NaN | NaN | NaN | NaN | NaN | NaN |
3660 | MIO_TKM | TOT | NL | BE | 998.0 | 1070.0 | 1048.0 | 950.0 | 1011.0 | 957.0 | ... | 1005.0 | 817.0 | 885.0 | 823.0 | 798.0 | 809.0 | 744.0 | 658.0 | 556.0 | 583.0 |
2112 | MIO_TKM | OWN | HR | PL | 16.0 | 38.0 | 8.0 | 8.0 | 42.0 | 1.0 | ... | NaN | NaN | NaN | NaN | NaN | NaN | NaN | NaN | NaN | NaN |
1974 | MIO_TKM | OWN | EU28 | DK | 82.0 | 57.0 | 59.0 | 58.0 | 42.0 | 78.0 | ... | NaN | NaN | NaN | NaN | NaN | NaN | NaN | NaN | NaN | NaN |
3482 | MIO_TKM | TOT | KZ | LV | 87.0 | 30.0 | 14.0 | 30.0 | 214.0 | 109.0 | ... | NaN | NaN | NaN | NaN | NaN | NaN | NaN | NaN | NaN | NaN |
1281 | MIO_TKM | HIRE | RU | SI | 164.0 | 113.0 | 71.0 | 116.0 | 225.0 | 176.0 | ... | NaN | NaN | NaN | NaN | NaN | NaN | NaN | NaN | NaN | NaN |
1249 | MIO_TKM | HIRE | RS_ME | LU | NaN | NaN | NaN | NaN | NaN | NaN | ... | NaN | NaN | NaN | NaN | NaN | NaN | NaN | NaN | NaN | NaN |
3717 | MIO_TKM | TOT | NO | UK | 5.0 | 46.0 | 2.0 | 1.0 | NaN | 0.0 | ... | 2.0 | 4.0 | 13.0 | 0.0 | 0.0 | 0.0 | 0.0 | 1.0 | 1.0 | 1.0 |
1270 | MIO_TKM | HIRE | RU | HR | 19.0 | NaN | NaN | 21.0 | 17.0 | 39.0 | ... | NaN | NaN | NaN | NaN | NaN | NaN | NaN | NaN | NaN | NaN |
10 rows × 42 columns
df_unload.sample(10)
unit | carriage | c_load | geo\time | 2019 | 2018 | 2017 | 2016 | 2015 | 2014 | ... | 1991 | 1990 | 1989 | 1988 | 1987 | 1986 | 1985 | 1984 | 1983 | 1982 | |
---|---|---|---|---|---|---|---|---|---|---|---|---|---|---|---|---|---|---|---|---|---|
2735 | MIO_TKM | TOT | EE | HU | NaN | NaN | 5.0 | NaN | 6.0 | 4.0 | ... | NaN | NaN | NaN | NaN | NaN | NaN | NaN | NaN | NaN | NaN |
3769 | MIO_TKM | TOT | UK | IE | 407.0 | 387.0 | 406.0 | 397.0 | 381.0 | 369.0 | ... | 135.0 | 181.0 | 185.0 | 167.0 | 159.0 | 134.0 | 107.0 | 90.0 | 103.0 | 76.0 |
2048 | MIO_TKM | OWN | LI | CZ | NaN | NaN | NaN | 22.0 | NaN | NaN | ... | NaN | NaN | NaN | NaN | NaN | NaN | NaN | NaN | NaN | NaN |
1330 | MIO_TKM | HIRE | UA | EU15 | NaN | NaN | NaN | NaN | NaN | NaN | ... | NaN | NaN | NaN | NaN | NaN | NaN | NaN | NaN | NaN | NaN |
1314 | MIO_TKM | HIRE | TR | LU | NaN | NaN | NaN | NaN | NaN | NaN | ... | NaN | NaN | NaN | NaN | NaN | NaN | NaN | NaN | NaN | NaN |
1348 | MIO_TKM | HIRE | UK | CH | NaN | 31.0 | NaN | NaN | 2.0 | NaN | ... | NaN | NaN | NaN | NaN | NaN | NaN | NaN | NaN | NaN | NaN |
2712 | MIO_TKM | TOT | EA | IE | NaN | NaN | NaN | NaN | NaN | NaN | ... | 266.0 | 311.0 | 355.0 | 260.0 | 251.0 | 272.0 | 253.0 | 172.0 | 189.0 | 142.0 |
932 | MIO_TKM | HIRE | MA | PT | NaN | NaN | NaN | NaN | NaN | NaN | ... | NaN | NaN | NaN | NaN | NaN | NaN | NaN | NaN | NaN | NaN |
38 | MIO_TKM | HIRE | AT | DK | NaN | 2.0 | 3.0 | 10.0 | 3.0 | NaN | ... | 0.0 | 0.0 | 0.0 | 0.0 | 0.0 | 0.0 | 0.0 | 0.0 | 0.0 | 0.0 |
2462 | MIO_TKM | TOT | AT | PT | 144.0 | 164.0 | 39.0 | 33.0 | 19.0 | 91.0 | ... | 21.0 | 25.0 | 12.0 | 52.0 | 19.0 | 0.0 | NaN | NaN | NaN | NaN |
10 rows × 42 columns
10.3. Clean Up#
The meaning of keys are illustrated here: https://ec.europa.eu/eurostat/cache/metadata/en/road_go_esms.htm
rename columns
df_load.rename(
columns={
"geo\\time": "origin",
"c_unload": "destination"
},
inplace=True
)
df_unload.rename(
columns={
"geo\\time": "destination",
"c_load": "origin"
},
inplace=True
)
for col in df_load.columns:
df_load.rename(
columns={
col: col.strip()
},
inplace=True
)
for col in df_unload.columns:
df_unload.rename(
columns={
col: col.strip()
},
inplace=True
)
df_load.replace({df_load["2019"].iloc[0], 1000})
unit | carriage | destination | origin | 2019 | 2018 | 2017 | 2016 | 2015 | 2014 | ... | 1991 | 1990 | 1989 | 1988 | 1987 | 1986 | 1985 | 1984 | 1983 | 1982 | |
---|---|---|---|---|---|---|---|---|---|---|---|---|---|---|---|---|---|---|---|---|---|
0 | MIO_TKM | HIRE | AD | CZ | NaN | NaN | NaN | NaN | NaN | NaN | ... | NaN | NaN | NaN | NaN | NaN | NaN | NaN | NaN | NaN | NaN |
1 | MIO_TKM | HIRE | AD | DE | NaN | 3.0 | NaN | NaN | NaN | NaN | ... | NaN | NaN | NaN | NaN | NaN | NaN | NaN | NaN | NaN | NaN |
2 | MIO_TKM | HIRE | AD | ES | 46.0 | 54.0 | 18.0 | 29.0 | 68.0 | 39.0 | ... | NaN | NaN | NaN | NaN | NaN | NaN | NaN | NaN | NaN | NaN |
3 | MIO_TKM | HIRE | AD | FR | 2.0 | 0.0 | NaN | NaN | NaN | NaN | ... | NaN | NaN | NaN | NaN | NaN | NaN | NaN | NaN | NaN | NaN |
4 | MIO_TKM | HIRE | AD | HU | NaN | NaN | NaN | NaN | 5.0 | NaN | ... | NaN | NaN | NaN | NaN | NaN | NaN | NaN | NaN | NaN | NaN |
... | ... | ... | ... | ... | ... | ... | ... | ... | ... | ... | ... | ... | ... | ... | ... | ... | ... | ... | ... | ... | ... |
4123 | MIO_TKM | TOT | WORLD | SE | 1255.0 | 1453.0 | 1791.0 | 1658.0 | 1828.0 | 1617.0 | ... | NaN | NaN | NaN | NaN | NaN | NaN | NaN | NaN | NaN | NaN |
4124 | MIO_TKM | TOT | WORLD | SI | 5618.0 | 5661.0 | 5152.0 | 4702.0 | 4771.0 | 4308.0 | ... | NaN | NaN | NaN | NaN | NaN | NaN | NaN | NaN | NaN | NaN |
4125 | MIO_TKM | TOT | WORLD | SK | 8472.0 | 8683.0 | 8290.0 | 8357.0 | 7902.0 | 6942.0 | ... | NaN | NaN | NaN | NaN | NaN | NaN | NaN | NaN | NaN | NaN |
4126 | MIO_TKM | TOT | WORLD | UK | 3073.0 | 3315.0 | 3072.0 | 2959.0 | 3487.0 | 3788.0 | ... | 5604.0 | 5413.0 | 4519.0 | 3900.0 | 3255.0 | 2110.0 | 2003.0 | 2073.0 | 2037.0 | 1767.0 |
4127 | MIO_TKM | TOT | XK | HR | 2.0 | 3.0 | 1.0 | 8.0 | 7.0 | 2.0 | ... | NaN | NaN | NaN | NaN | NaN | NaN | NaN | NaN | NaN | NaN |
4128 rows × 42 columns
msno.matrix(df_load)
<AxesSubplot:>
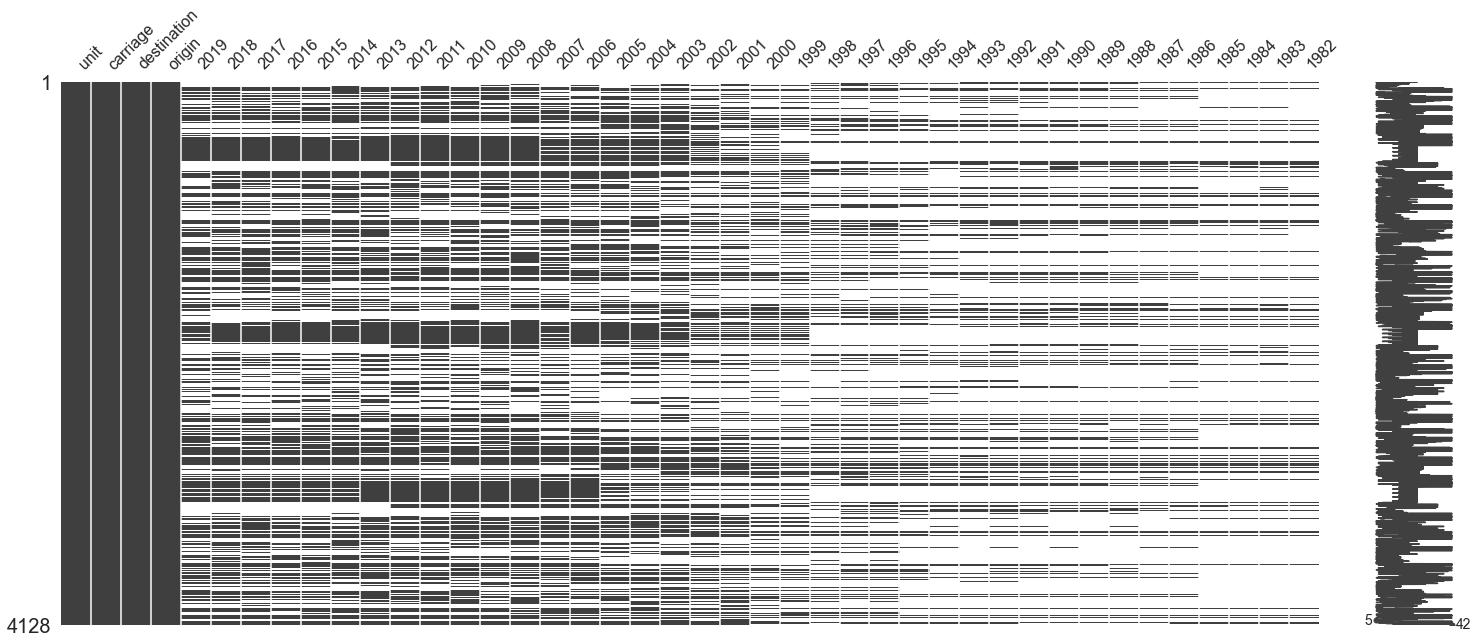
msno.matrix(df_unload)
<AxesSubplot:>
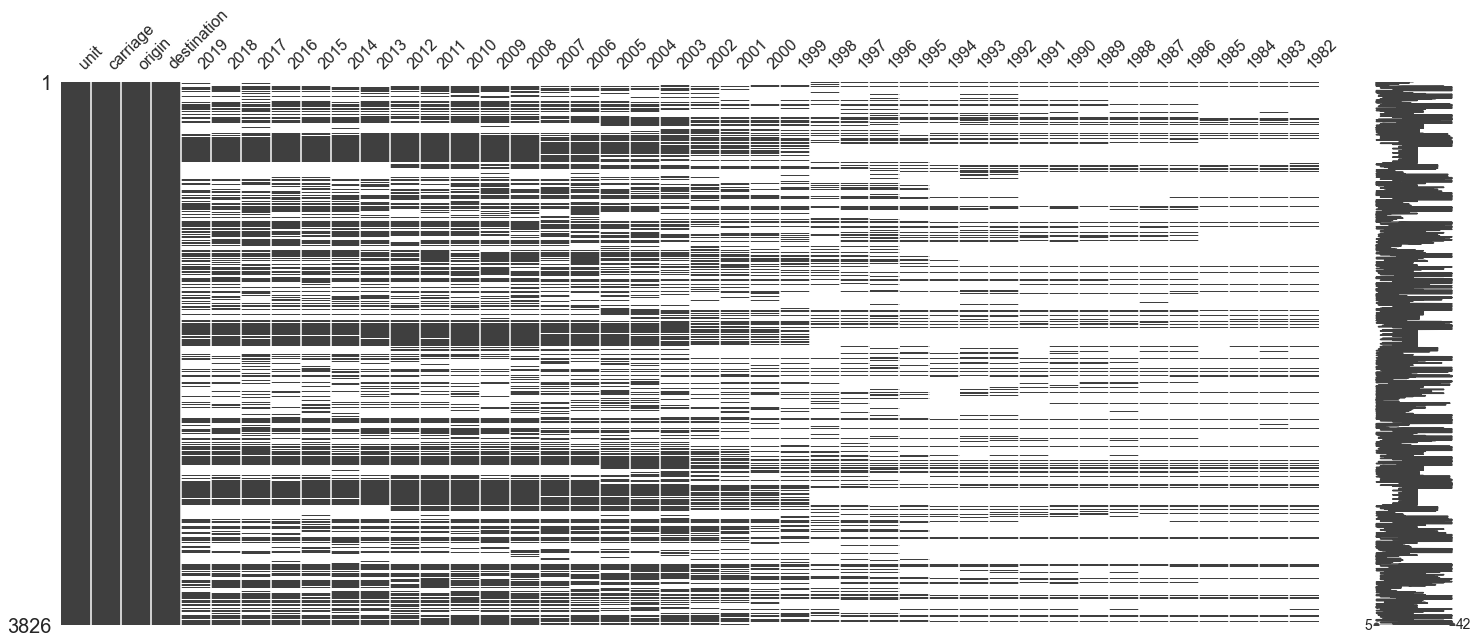
Here is brief look into the data
df_load.columns
Index(['unit', 'carriage', 'destination', 'origin', '2019', '2018', '2017',
'2016', '2015', '2014', '2013', '2012', '2011', '2010', '2009', '2008',
'2007', '2006', '2005', '2004', '2003', '2002', '2001', '2000', '1999',
'1998', '1997', '1996', '1995', '1994', '1993', '1992', '1991', '1990',
'1989', '1988', '1987', '1986', '1985', '1984', '1983', '1982'],
dtype='object')
df_load.unit.unique()
array(['MIO_TKM'], dtype=object)
df_load.carriage.unique()
array(['HIRE', 'NOT_SPEC', 'OWN', 'TOT'], dtype=object)
HIRE
: hire or rewardOWN
:
df_load.destination.unique()
array(['AD', 'AF', 'AFR_N', 'AL', 'AM', 'AT', 'AZ', 'BA', 'BE', 'BG',
'BH', 'BY', 'CH', 'CY', 'CZ', 'CZ_SK', 'DE', 'DK', 'DZ', 'EA',
'EE', 'EEA_X_LI', 'EG', 'EH', 'EL', 'ES', 'EU15', 'EU25',
'EU27_2007', 'EU27_2020', 'EU28', 'EUR_OTH', 'EXT_EU15', 'EX_DD',
'EX_SU', 'EX_YU', 'FI', 'FR', 'GE', 'GI', 'HR', 'HU', 'IE', 'IL',
'IQ', 'IR', 'IS', 'IT', 'JO', 'KG', 'KZ', 'LB', 'LI', 'LT', 'LU',
'LV', 'MA', 'MC', 'MD', 'ME', 'MK', 'MN', 'MT', 'NE', 'NL', 'NO',
'OTH', 'PL', 'PT', 'RO', 'RS', 'RS_ME', 'RU', 'SE', 'SI', 'SK',
'SL', 'SM', 'SY', 'TJ', 'TM', 'TN', 'TR', 'UA', 'UK', 'UNK', 'US',
'UZ', 'VA', 'WORLD', 'XK'], dtype=object)
df_load.origin.unique()
array(['CZ', 'DE', 'ES', 'FR', 'HU', 'LU', 'NL', 'PT', 'UK', 'LV', 'DK',
'EL', 'EU15', 'IE', 'IT', 'AT', 'BE', 'BG', 'CY', 'FI', 'HR', 'RO',
'SE', 'SI', 'LT', 'PL', 'SK', 'CH', 'EE', 'LI', 'NO'], dtype=object)
For the purpose of demonstration, we will only use part of the data.
We will only look at 2019
We will only look at HIRE
lunl_c_origin = [
i for i in set.intersection(
set(df_load.origin.unique()),
set(df_load.destination.unique())
) if len(i) == 2
]
df_load_selected = df_load[['unit', 'carriage', 'destination', 'origin', '2019', '2018', '2017', '2016', '2015', '2014', '2013', '2012', '2011', '2010']]
df_load_selected_com = df_load_selected.loc[
(
df_load_selected.carriage == "HIRE"
) & (
df_load_selected.destination.isin(lunl_c_origin)
) & (
df_load_selected.origin.isin(lunl_c_origin)
)
]
df_load_selected_com#.sample(10)
unit | carriage | destination | origin | 2019 | 2018 | 2017 | 2016 | 2015 | 2014 | 2013 | 2012 | 2011 | 2010 | |
---|---|---|---|---|---|---|---|---|---|---|---|---|---|---|
48 | MIO_TKM | HIRE | AT | BE | 53.0 | 85.0 | 53.0 | 55.0 | 102.0 | 11.0 | 19.0 | 21.0 | 19.0 | 18.0 |
49 | MIO_TKM | HIRE | AT | BG | 126.0 | 97.0 | 229.0 | 119.0 | 147.0 | 95.0 | 220.0 | 131.0 | 66.0 | 46.0 |
50 | MIO_TKM | HIRE | AT | CH | 9.0 | 20.0 | 20.0 | 16.0 | 30.0 | 46.0 | 53.0 | 37.0 | 64.0 | 47.0 |
51 | MIO_TKM | HIRE | AT | CY | 0.0 | 0.0 | 1.0 | 1.0 | 0.0 | 0.0 | 0.0 | 1.0 | 0.0 | 1.0 |
52 | MIO_TKM | HIRE | AT | CZ | 454.0 | 478.0 | 678.0 | 577.0 | 700.0 | 779.0 | 722.0 | 651.0 | 572.0 | 589.0 |
... | ... | ... | ... | ... | ... | ... | ... | ... | ... | ... | ... | ... | ... | ... |
1468 | MIO_TKM | HIRE | UK | PT | 460.0 | 380.0 | 617.0 | 742.0 | 516.0 | 270.0 | 403.0 | 366.0 | 408.0 | 236.0 |
1469 | MIO_TKM | HIRE | UK | RO | 211.0 | 371.0 | 314.0 | 390.0 | 420.0 | 370.0 | 281.0 | 280.0 | 364.0 | 514.0 |
1470 | MIO_TKM | HIRE | UK | SE | NaN | NaN | 3.0 | NaN | NaN | NaN | 4.0 | 5.0 | 12.0 | 1.0 |
1471 | MIO_TKM | HIRE | UK | SI | 121.0 | 176.0 | 135.0 | 193.0 | 211.0 | 157.0 | 221.0 | 144.0 | 197.0 | 186.0 |
1472 | MIO_TKM | HIRE | UK | SK | 353.0 | 421.0 | 293.0 | 441.0 | 278.0 | 233.0 | 421.0 | 234.0 | 476.0 | 208.0 |
788 rows × 14 columns
years = ['2019', '2018', '2017', '2016', '2015', '2014', '2013', '2012', '2011', '2010']
df_unload_19 = df_unload[['unit', 'carriage', 'origin', 'destination'] + years]
df_unload_19_com = df_unload_19.loc[
(
df_unload_19.carriage == "HIRE"
) & (
df_unload_19.origin.apply(lambda x: len(x)==2)
)
]
df_unload_19_com.sample(10)
unit | carriage | origin | destination | 2019 | 2018 | 2017 | 2016 | 2015 | 2014 | 2013 | 2012 | 2011 | 2010 | |
---|---|---|---|---|---|---|---|---|---|---|---|---|---|---|
83 | MIO_TKM | HIRE | BA | UK | NaN | NaN | NaN | NaN | NaN | NaN | NaN | NaN | NaN | NaN |
1124 | MIO_TKM | HIRE | RO | FR | NaN | NaN | NaN | NaN | NaN | 7.0 | NaN | NaN | NaN | NaN |
54 | MIO_TKM | HIRE | AT | NO | NaN | NaN | 1.0 | NaN | NaN | NaN | NaN | NaN | NaN | 1.0 |
788 | MIO_TKM | HIRE | IE | SI | NaN | NaN | NaN | NaN | NaN | NaN | NaN | NaN | NaN | NaN |
1343 | MIO_TKM | HIRE | UA | SK | 6.0 | 6.0 | 5.0 | 10.0 | 91.0 | 28.0 | 44.0 | 17.0 | 39.0 | 8.0 |
1286 | MIO_TKM | HIRE | SL | EL | NaN | NaN | NaN | NaN | NaN | NaN | NaN | NaN | NaN | NaN |
898 | MIO_TKM | HIRE | LU | LU | NaN | NaN | NaN | NaN | NaN | NaN | NaN | NaN | NaN | NaN |
796 | MIO_TKM | HIRE | IR | NL | NaN | NaN | NaN | NaN | NaN | NaN | NaN | NaN | NaN | NaN |
707 | MIO_TKM | HIRE | GE | PL | NaN | NaN | NaN | 51.0 | NaN | NaN | NaN | NaN | NaN | NaN |
398 | MIO_TKM | HIRE | ES | DE | 378.0 | 344.0 | 464.0 | 303.0 | 538.0 | 535.0 | 586.0 | 814.0 | 950.0 | 1288.0 |
df_load_selected.to_csv("assets/road-freight-eurostats/eurostats_load_selected.csv")
11. Construct the Matrix#
df_lunl = df_load_selected_com.pivot(index='origin', columns='destination', values='2019')
df_lunl
destination | AT | BE | BG | CH | CY | CZ | DE | DK | EE | EL | ... | LV | NL | NO | PL | PT | RO | SE | SI | SK | UK |
---|---|---|---|---|---|---|---|---|---|---|---|---|---|---|---|---|---|---|---|---|---|
origin | |||||||||||||||||||||
AT | NaN | 55.0 | 21.0 | 98.0 | NaN | 42.0 | 1404.0 | 18.0 | NaN | 31.0 | ... | NaN | 62.0 | 17.0 | 10.0 | NaN | NaN | 45.0 | 27.0 | 33.0 | 36.0 |
BE | 53.0 | NaN | 15.0 | 77.0 | NaN | 11.0 | 1102.0 | 23.0 | NaN | NaN | ... | NaN | 807.0 | NaN | 36.0 | 31.0 | NaN | NaN | 5.0 | NaN | 152.0 |
BG | 126.0 | 24.0 | NaN | NaN | NaN | 44.0 | 382.0 | 22.0 | NaN | 461.0 | ... | NaN | 77.0 | NaN | 144.0 | 36.0 | 297.0 | 107.0 | 18.0 | 28.0 | 45.0 |
CH | 9.0 | 22.0 | NaN | NaN | NaN | NaN | 191.0 | NaN | NaN | NaN | ... | NaN | 13.0 | NaN | NaN | NaN | NaN | NaN | NaN | NaN | 20.0 |
CY | 0.0 | 0.0 | 0.0 | 0.0 | NaN | 0.0 | 1.0 | 2.0 | NaN | 1.0 | ... | 0.0 | 1.0 | NaN | 0.0 | 0.0 | 0.0 | 1.0 | NaN | 0.0 | 9.0 |
CZ | 454.0 | 227.0 | 24.0 | 84.0 | NaN | NaN | 2317.0 | 58.0 | NaN | 37.0 | ... | NaN | 304.0 | 45.0 | 201.0 | 26.0 | 17.0 | 73.0 | 33.0 | 631.0 | 137.0 |
DE | 2050.0 | 1981.0 | 8.0 | 1752.0 | NaN | 443.0 | NaN | 1257.0 | NaN | 13.0 | ... | NaN | 2741.0 | 106.0 | 432.0 | 306.0 | 32.0 | 270.0 | 25.0 | 59.0 | 689.0 |
DK | 47.0 | 2.0 | NaN | 3.0 | NaN | NaN | 344.0 | NaN | NaN | NaN | ... | NaN | 79.0 | 400.0 | 45.0 | NaN | NaN | 643.0 | NaN | NaN | 65.0 |
EE | 11.0 | NaN | NaN | NaN | NaN | NaN | 194.0 | 22.0 | NaN | NaN | ... | 108.0 | 54.0 | 15.0 | 41.0 | NaN | NaN | 88.0 | NaN | NaN | NaN |
EL | 282.0 | 150.0 | 95.0 | 12.0 | NaN | 155.0 | 2358.0 | 95.0 | NaN | NaN | ... | NaN | 533.0 | NaN | 156.0 | 83.0 | 141.0 | 72.0 | NaN | 39.0 | 289.0 |
ES | 459.0 | 1511.0 | 39.0 | 768.0 | NaN | 226.0 | 8339.0 | 543.0 | NaN | 27.0 | ... | NaN | 3586.0 | 166.0 | 451.0 | 3140.0 | 13.0 | 366.0 | NaN | 45.0 | 4054.0 |
FI | NaN | NaN | NaN | NaN | NaN | NaN | 5.0 | NaN | NaN | NaN | ... | 17.0 | NaN | 120.0 | NaN | NaN | NaN | 692.0 | NaN | NaN | NaN |
FR | 28.0 | 1203.0 | NaN | 355.0 | NaN | 5.0 | 701.0 | 6.0 | NaN | NaN | ... | NaN | 254.0 | NaN | 51.0 | 1.0 | NaN | NaN | NaN | NaN | 266.0 |
HR | 172.0 | 96.0 | 1.0 | 24.0 | NaN | 51.0 | 432.0 | 22.0 | NaN | NaN | ... | NaN | 68.0 | NaN | 30.0 | NaN | 21.0 | 13.0 | 184.0 | 21.0 | 58.0 |
HU | 694.0 | 225.0 | 33.0 | 103.0 | NaN | 345.0 | 2203.0 | 75.0 | 12.0 | 38.0 | ... | 11.0 | 279.0 | 23.0 | 149.0 | 39.0 | 231.0 | 30.0 | 141.0 | 243.0 | 273.0 |
IE | NaN | 11.0 | NaN | 12.0 | NaN | NaN | 44.0 | NaN | NaN | NaN | ... | NaN | 67.0 | NaN | NaN | 13.0 | 10.0 | 15.0 | NaN | NaN | 512.0 |
IT | 294.0 | 292.0 | NaN | 659.0 | NaN | 7.0 | 1999.0 | NaN | NaN | 31.0 | ... | NaN | 387.0 | NaN | 28.0 | 67.0 | 8.0 | 133.0 | 35.0 | 10.0 | 308.0 |
LI | NaN | NaN | NaN | NaN | NaN | NaN | NaN | NaN | NaN | NaN | ... | NaN | NaN | NaN | NaN | NaN | NaN | NaN | NaN | NaN | NaN |
LT | 105.0 | 88.0 | 7.0 | 43.0 | NaN | 148.0 | 982.0 | 145.0 | 167.0 | 21.0 | ... | 375.0 | 272.0 | 125.0 | 252.0 | 46.0 | 30.0 | 177.0 | 38.0 | 28.0 | 307.0 |
LU | 5.0 | 105.0 | NaN | 5.0 | NaN | NaN | 148.0 | 1.0 | NaN | NaN | ... | NaN | 58.0 | 0.0 | 2.0 | NaN | NaN | NaN | NaN | NaN | 3.0 |
LV | 43.0 | 169.0 | NaN | 13.0 | NaN | 59.0 | 652.0 | 7.0 | 317.0 | 2.0 | ... | NaN | 212.0 | 86.0 | 36.0 | NaN | 20.0 | 90.0 | 63.0 | NaN | 5.0 |
NL | 99.0 | 3092.0 | NaN | 329.0 | NaN | 43.0 | 5428.0 | 393.0 | 15.0 | 50.0 | ... | NaN | NaN | 194.0 | 41.0 | 13.0 | 15.0 | 723.0 | 8.0 | 7.0 | 769.0 |
NO | NaN | 15.0 | NaN | 1.0 | NaN | NaN | 67.0 | 69.0 | NaN | NaN | ... | NaN | 37.0 | NaN | NaN | NaN | NaN | 702.0 | NaN | NaN | NaN |
PL | 1203.0 | 2799.0 | 151.0 | 270.0 | NaN | 2879.0 | 22471.0 | 1566.0 | 191.0 | 406.0 | ... | 274.0 | 3701.0 | 203.0 | NaN | 219.0 | 1617.0 | 1779.0 | 668.0 | 1731.0 | 4602.0 |
PT | 59.0 | 212.0 | NaN | 162.0 | NaN | 91.0 | 854.0 | 26.0 | NaN | NaN | ... | NaN | 202.0 | 7.0 | 61.0 | NaN | NaN | 60.0 | 15.0 | 30.0 | 460.0 |
RO | 404.0 | 654.0 | 64.0 | 66.0 | NaN | 249.0 | 2152.0 | 93.0 | NaN | 150.0 | ... | NaN | 424.0 | 10.0 | 325.0 | 18.0 | NaN | 156.0 | 43.0 | 126.0 | 211.0 |
SE | NaN | 14.0 | NaN | 2.0 | NaN | NaN | 181.0 | 36.0 | 1.0 | 16.0 | ... | NaN | 37.0 | 646.0 | 1.0 | NaN | NaN | NaN | NaN | NaN | NaN |
SI | 477.0 | 178.0 | NaN | 48.0 | NaN | 104.0 | 1248.0 | 55.0 | NaN | 63.0 | ... | 13.0 | 214.0 | 36.0 | 121.0 | 16.0 | 25.0 | 53.0 | NaN | 65.0 | 121.0 |
SK | 701.0 | 252.0 | 17.0 | 170.0 | NaN | 982.0 | 2263.0 | 62.0 | NaN | NaN | ... | 0.0 | 247.0 | 6.0 | 167.0 | NaN | 163.0 | 57.0 | 81.0 | NaN | 353.0 |
UK | 14.0 | 271.0 | NaN | 32.0 | NaN | 8.0 | 274.0 | 8.0 | NaN | NaN | ... | NaN | 226.0 | 3.0 | 12.0 | 9.0 | NaN | 15.0 | NaN | 13.0 | NaN |
30 rows × 30 columns
fig, ax = plt.subplots(figsize=(30, 30))
sns.heatmap(
df_lunl, annot=True, fmt="0.1f", linewidths=.5, ax=ax
)
<AxesSubplot:xlabel='destination', ylabel='origin'>
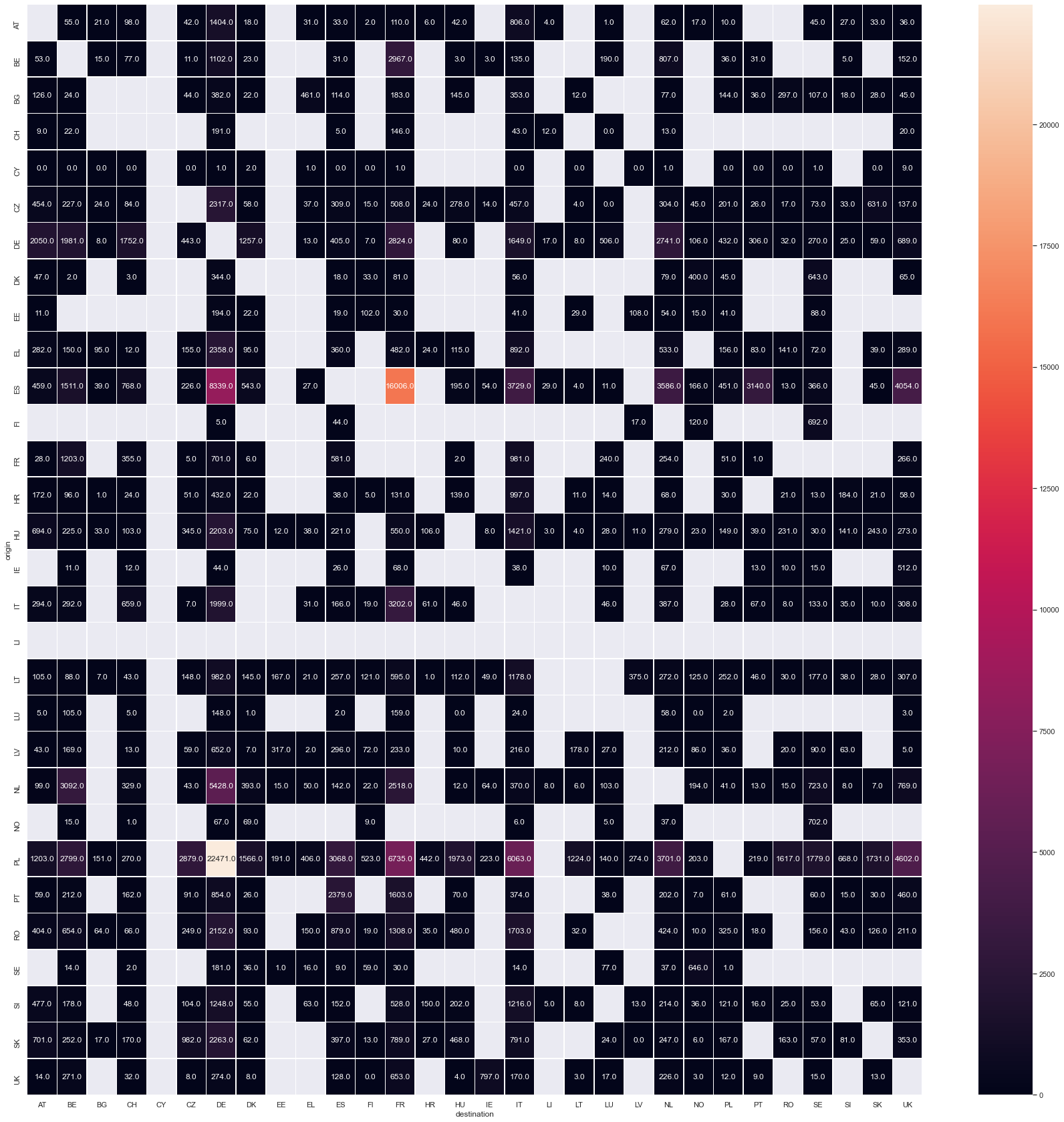
df_load_selected_com.head()
unit | carriage | destination | origin | 2019 | 2018 | 2017 | 2016 | 2015 | 2014 | 2013 | 2012 | 2011 | 2010 | |
---|---|---|---|---|---|---|---|---|---|---|---|---|---|---|
48 | MIO_TKM | HIRE | AT | BE | 53.0 | 85.0 | 53.0 | 55.0 | 102.0 | 11.0 | 19.0 | 21.0 | 19.0 | 18.0 |
49 | MIO_TKM | HIRE | AT | BG | 126.0 | 97.0 | 229.0 | 119.0 | 147.0 | 95.0 | 220.0 | 131.0 | 66.0 | 46.0 |
50 | MIO_TKM | HIRE | AT | CH | 9.0 | 20.0 | 20.0 | 16.0 | 30.0 | 46.0 | 53.0 | 37.0 | 64.0 | 47.0 |
51 | MIO_TKM | HIRE | AT | CY | 0.0 | 0.0 | 1.0 | 1.0 | 0.0 | 0.0 | 0.0 | 1.0 | 0.0 | 1.0 |
52 | MIO_TKM | HIRE | AT | CZ | 454.0 | 478.0 | 678.0 | 577.0 | 700.0 | 779.0 | 722.0 | 651.0 | 572.0 | 589.0 |
D = nx.from_pandas_edgelist(df_load_selected_com, "origin", "destination", edge_attr="2019", create_using=nx.DiGraph)
fig, ax = plt.subplots(figsize=(20, 20))
nx_draw_options = {
"node_color": "r",
"node_size": 500,
"alpha": 0.8,
"edge_color": "b"
}
nx.draw(D, pos=nx.spring_layout(D, k=0.9, iterations=50), ax=ax, with_labels=True, **nx_draw_options)
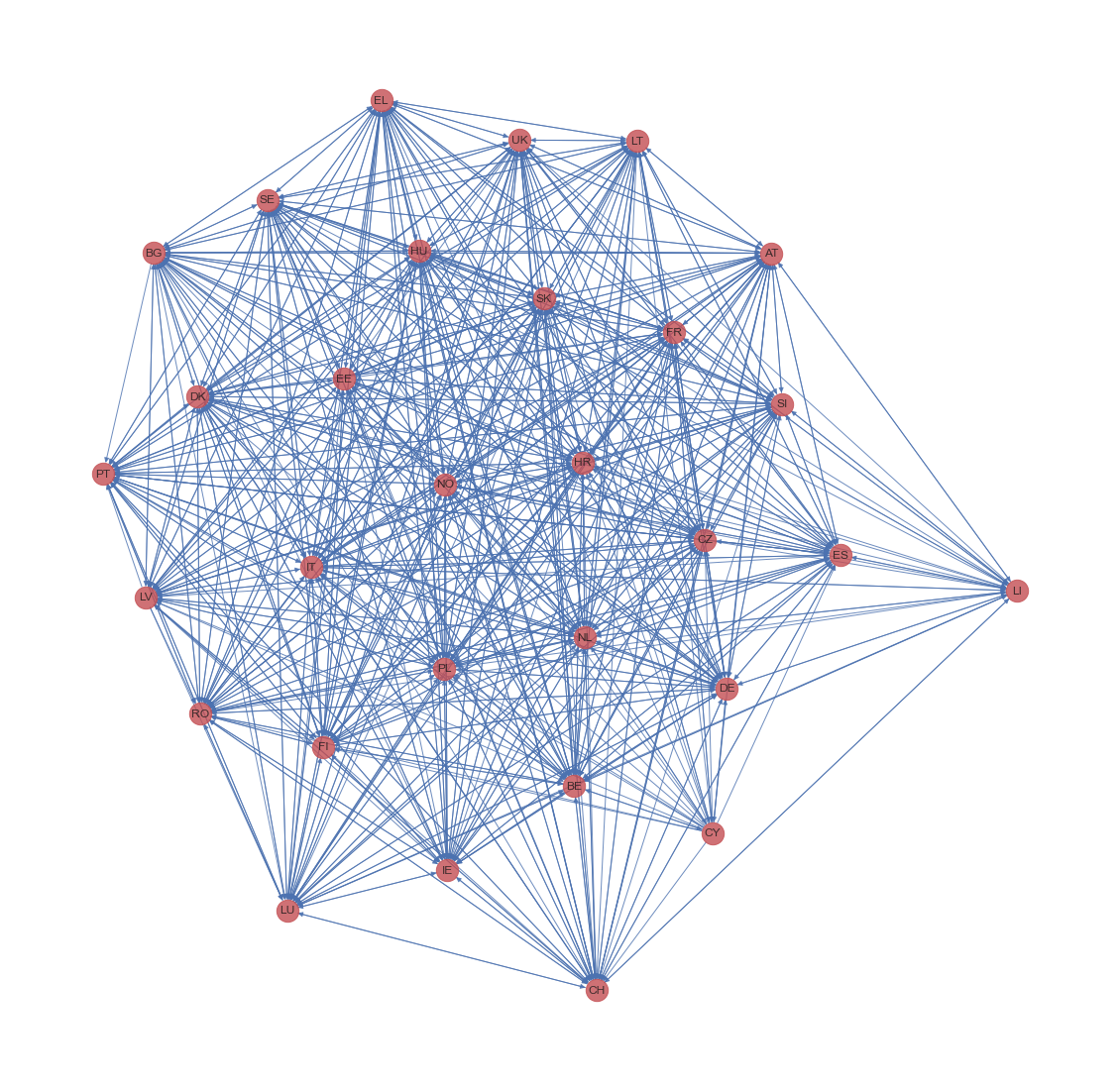
11.1. Build a Minimal Network#
lunl_minimal = ["AT", "CH", "DE"]
df_lunl_minimal = {}
for year in years:
df_lunl_minimal[year] = df_load_selected_com.pivot(index='origin', columns='destination', values=year).loc[lunl_minimal, lunl_minimal]
df_lunl_minimal;
fig, ax = plt.subplots(nrows=2, ncols=2, figsize=(12, 10))
sns.heatmap(
df_lunl_minimal["2019"], annot=True, fmt="0.1f", linewidths=.5, ax=ax[0,0]
)
sns.heatmap(
df_lunl_minimal["2018"], annot=True, fmt="0.1f", linewidths=.5, ax=ax[0,1]
)
sns.heatmap(
df_lunl_minimal["2017"], annot=True, fmt="0.1f", linewidths=.5, ax=ax[1,0]
)
sns.heatmap(
df_lunl_minimal["2016"], annot=True, fmt="0.1f", linewidths=.5, ax=ax[1,1]
)
<AxesSubplot:xlabel='destination', ylabel='origin'>
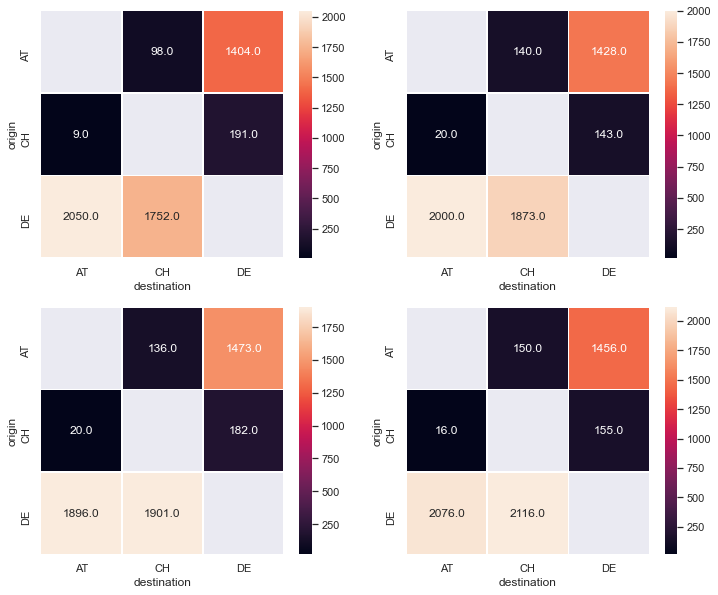
D_minimal = nx.DiGraph(df_lunl_minimal["2019"])
fig, ax = plt.subplots(figsize=(10, 10))
nx_draw_options = {
"node_color": "r",
"node_size": 500,
"alpha": 0.8,
"edge_color": "b"
}
nx.draw(D_minimal, pos=nx.spring_layout(D, k=0.4, iterations=10), ax=ax, with_labels=True, **nx_draw_options)
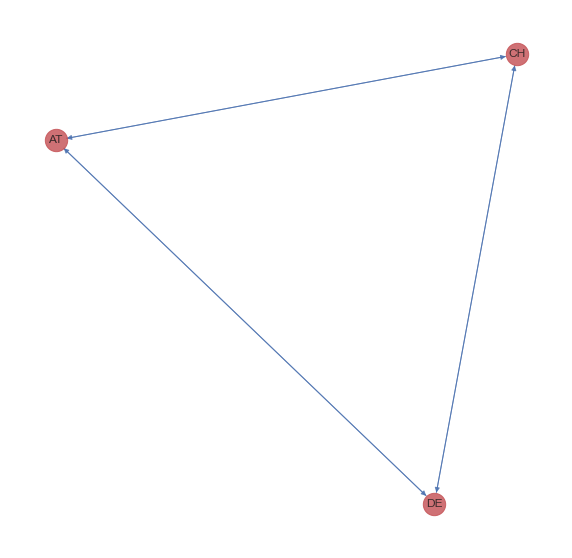
12. Naive Model#
A naive idea is that the demand on the lane depends on the total demand of the origin and the total demand of the destination.
def naive_model_kpq(o_t, d_t, data):
k_pq = {}
for p in o_t:
for q in d_t:
if p != q:
k_pq[(p,q)] = data[p][q] / (o_t[p] * d_t[q])
return k_pq
def naive_model(p, q, k_pq, o_t, d_t):
g_pq = k_pq[(p,q)] * (o_t[p] * d_t[q])
return g_pq
df_lunl_minimal["2019"].to_dict()
{'AT': {'AT': nan, 'CH': 9.0, 'DE': 2050.0},
'CH': {'AT': 98.0, 'CH': nan, 'DE': 1752.0},
'DE': {'AT': 1404.0, 'CH': 191.0, 'DE': nan}}
total_demand_origin["2019"]
{'AT': 1502.0, 'CH': 200.0, 'DE': 3802.0}
total_demand_dest["2019"]
{'AT': 2059.0, 'CH': 1850.0, 'DE': 1595.0}
naive_kpqs = {}
for year in years:
naive_kpqs[year] = naive_model_kpq(total_demand_origin[year], total_demand_dest[year], df_lunl_minimal[year].to_dict())
naive_kpqs[year]
{('AT', 'CH'): 8.37794141501648e-06,
('AT', 'DE'): 0.00046207319268525015,
('CH', 'AT'): 0.00020008003201280514,
('CH', 'DE'): 0.0019352568613652356,
('DE', 'AT'): 0.00016255303019258526,
('DE', 'CH'): 3.604375661642385e-05}
fig, ax = plt.subplots(figsize=(10, 6.18))
for key in naive_kpqs[year]:
ax.plot(
naive_kpqs.keys(),
[val[key] for idx, val in naive_kpqs.items()],
"-",
marker="o",
label=key
)
ax.set_yscale("log")
plt.legend()
<matplotlib.legend.Legend at 0x7fadfa5c7e90>
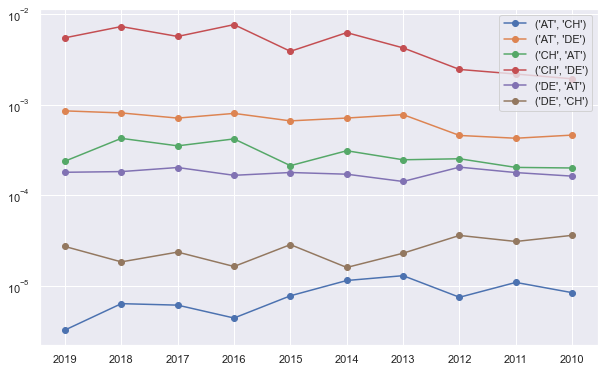
total_demand_origin
{'2019': {'AT': 1502.0, 'CH': 200.0, 'DE': 3802.0},
'2018': {'AT': 1568.0, 'CH': 163.0, 'DE': 3873.0},
'2017': {'AT': 1609.0, 'CH': 202.0, 'DE': 3797.0},
'2016': {'AT': 1606.0, 'CH': 171.0, 'DE': 4192.0},
'2015': {'AT': 1681.0, 'CH': 306.0, 'DE': 4205.0},
'2014': {'AT': 1685.0, 'CH': 210.0, 'DE': 4310.0},
'2013': {'AT': 1661.0, 'CH': 310.0, 'DE': 4545.0},
'2012': {'AT': 2042.0, 'CH': 410.0, 'DE': 4268.0},
'2011': {'AT': 2292.0, 'CH': 439.0, 'DE': 4740.0},
'2010': {'AT': 2253.0, 'CH': 476.0, 'DE': 4780.0}}
fig, ax = plt.subplots(figsize=(10, 6.18))
for key in total_demand_origin["2019"]:
ax.plot(
total_demand_origin.keys(),
[val[key] for idx, val in total_demand_origin.items()],
"-",
marker="o",
label=key
)
ax.set_yscale("log")
plt.legend()
<matplotlib.legend.Legend at 0x7fadfa269810>
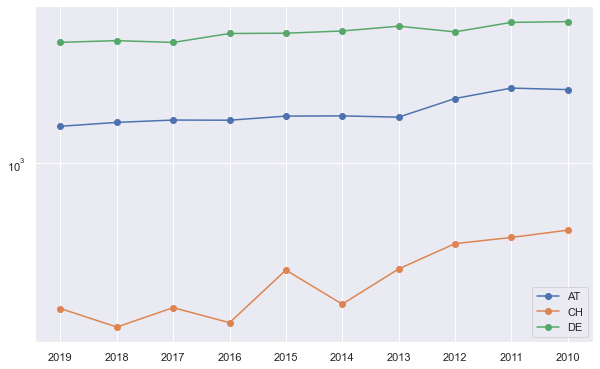
fig, ax = plt.subplots(figsize=(10, 6.18))
for key in total_demand_dest["2019"]:
ax.plot(
total_demand_origin.keys(),
[val[key] for idx, val in total_demand_dest.items()],
"-",
marker="o",
label=key
)
ax.set_yscale("log")
plt.legend()
<matplotlib.legend.Legend at 0x7fadfb5bce90>
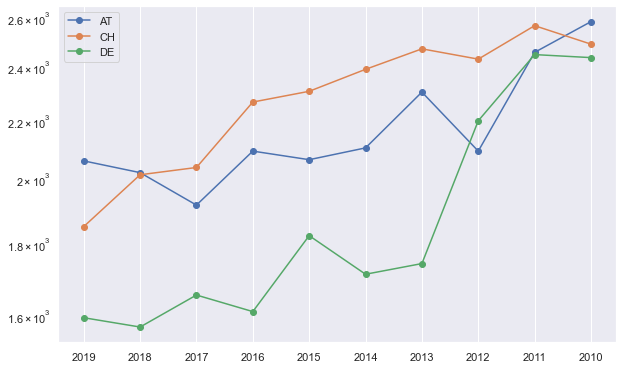
validate_year = "2018"
naive_kpq = naive_kpqs["2017"]
for i_o in lunl_minimal:
for i_d in lunl_minimal:
if i_o != i_d:
print(
f"({i_o}, {i_d}):",
naive_model(i_o, i_d, naive_kpq, total_demand_origin[validate_year], total_demand_dest[validate_year])
)
(AT, CH): 19.260730555573353
(AT, DE): 1753.906890057625
(CH, AT): 115.69937369519833
(CH, DE): 1456.1178935718347
(DE, AT): 1584.0376921017196
(DE, CH): 183.45562557526424
df_lunl_minimal[validate_year].to_dict()
{'AT': {'AT': nan, 'CH': 20.0, 'DE': 2000.0},
'CH': {'AT': 140.0, 'CH': nan, 'DE': 1873.0},
'DE': {'AT': 1428.0, 'CH': 143.0, 'DE': nan}}
df_lunl_minimal["2017"].to_dict()
{'AT': {'AT': nan, 'CH': 20.0, 'DE': 1896.0},
'CH': {'AT': 136.0, 'CH': nan, 'DE': 1901.0},
'DE': {'AT': 1473.0, 'CH': 182.0, 'DE': nan}}
df_lunl_minimal["2018"] - df_lunl_minimal["2017"]
destination | AT | CH | DE |
---|---|---|---|
origin | |||
AT | NaN | 4.0 | -45.0 |
CH | 0.0 | NaN | -39.0 |
DE | 104.0 | -28.0 | NaN |
total_demand_origin["2015"]
{'AT': 1681.0, 'CH': 306.0, 'DE': 4205.0}
total_demand_dest["2015"]
{'AT': 2063.0, 'CH': 2306.0, 'DE': 1823.0}
mass_multiplications = {}
for year in years:
df_mass_mul_year = pd.DataFrame(
np.outer(
list(total_demand_origin[year].values()),
list(total_demand_dest[year].values())
),
columns=total_demand_origin[year].keys()
)
df_mass_mul_year.index = total_demand_origin[year].keys()
mass_multiplications[year] = df_mass_mul_year.copy()
mass_multiplications["2018"]
AT | CH | DE | |
---|---|---|---|
AT | 3167360.0 | 3156384.0 | 2463328.0 |
CH | 329260.0 | 328119.0 | 256073.0 |
DE | 7823460.0 | 7796349.0 | 6084483.0 |
year_1 = "2014"
year_2 = "2015"
df_mass_mul_diff = mass_multiplications[year_2] - mass_multiplications[year_1]
df_mass_mul_diff[df_mass_mul_diff >0] = 1
df_mass_mul_diff[df_mass_mul_diff <0] = -1
df_mass_mul_diff.values[[np.arange(df_mass_mul_diff.shape[0])]*2] = np.nan
df_demand_diff = df_lunl_minimal[year_2] - df_lunl_minimal[year_1]
df_demand_diff[df_demand_diff >0] = 1
df_demand_diff[df_demand_diff <0] = -1
fig, ax = plt.subplots(ncols=2, figsize=(10,5))
ax[0].matshow(df_mass_mul_diff)
ax[1].matshow(df_demand_diff)
/Users/leima/anaconda3/envs/theflow-code/lib/python3.7/site-packages/ipykernel_launcher.py:7: FutureWarning: Using a non-tuple sequence for multidimensional indexing is deprecated; use `arr[tuple(seq)]` instead of `arr[seq]`. In the future this will be interpreted as an array index, `arr[np.array(seq)]`, which will result either in an error or a different result.
import sys
<matplotlib.image.AxesImage at 0x7fadfb8cf850>
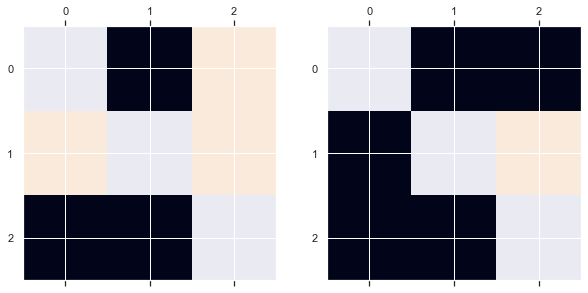
df_demand_diff
destination | AT | CH | DE |
---|---|---|---|
origin | |||
AT | NaN | -1.0 | -1.0 |
CH | -1.0 | NaN | 1.0 |
DE | -1.0 | -1.0 | NaN |
13. Four Stage Model#
df_load_selected_com_minimal = df_load_selected_com.loc[
(
df_load_selected_com.origin.isin(lunl_minimal)
) & (
df_load_selected_com.destination.isin(lunl_minimal)
)
]
df_load_selected_com_minimal
unit | carriage | destination | origin | 2019 | 2018 | 2017 | 2016 | 2015 | 2014 | 2013 | 2012 | 2011 | 2010 | |
---|---|---|---|---|---|---|---|---|---|---|---|---|---|---|
50 | MIO_TKM | HIRE | AT | CH | 9.0 | 20.0 | 20.0 | 16.0 | 30.0 | 46.0 | 53.0 | 37.0 | 64.0 | 47.0 |
53 | MIO_TKM | HIRE | AT | DE | 2050.0 | 2000.0 | 1896.0 | 2076.0 | 2033.0 | 2057.0 | 2250.0 | 2055.0 | 2393.0 | 2536.0 |
186 | MIO_TKM | HIRE | CH | AT | 98.0 | 140.0 | 136.0 | 150.0 | 134.0 | 137.0 | 176.0 | 217.0 | 219.0 | 246.0 |
191 | MIO_TKM | HIRE | CH | DE | 1752.0 | 1873.0 | 1901.0 | 2116.0 | 2172.0 | 2253.0 | 2295.0 | 2213.0 | 2347.0 | 2244.0 |
261 | MIO_TKM | HIRE | DE | AT | 1404.0 | 1428.0 | 1473.0 | 1456.0 | 1547.0 | 1548.0 | 1485.0 | 1825.0 | 2073.0 | 2007.0 |
264 | MIO_TKM | HIRE | DE | CH | 191.0 | 143.0 | 182.0 | 155.0 | 276.0 | 164.0 | 257.0 | 373.0 | 375.0 | 429.0 |
267 | MIO_TKM | HIRE | DE | DE | NaN | NaN | NaN | NaN | NaN | NaN | NaN | NaN | NaN | NaN |
df_load_selected_com_minimal_origin = df_load_selected_com_minimal.groupby("origin").sum()
df_load_selected_com_minimal_origin
2019 | 2018 | 2017 | 2016 | 2015 | 2014 | 2013 | 2012 | 2011 | 2010 | |
---|---|---|---|---|---|---|---|---|---|---|
origin | ||||||||||
AT | 1502.0 | 1568.0 | 1609.0 | 1606.0 | 1681.0 | 1685.0 | 1661.0 | 2042.0 | 2292.0 | 2253.0 |
CH | 200.0 | 163.0 | 202.0 | 171.0 | 306.0 | 210.0 | 310.0 | 410.0 | 439.0 | 476.0 |
DE | 3802.0 | 3873.0 | 3797.0 | 4192.0 | 4205.0 | 4310.0 | 4545.0 | 4268.0 | 4740.0 | 4780.0 |
df_load_selected_com_minimal_dest = df_load_selected_com_minimal.groupby("destination").sum()
df_load_selected_com_minimal_dest
2019 | 2018 | 2017 | 2016 | 2015 | 2014 | 2013 | 2012 | 2011 | 2010 | |
---|---|---|---|---|---|---|---|---|---|---|
destination | ||||||||||
AT | 2059.0 | 2020.0 | 1916.0 | 2092.0 | 2063.0 | 2103.0 | 2303.0 | 2092.0 | 2457.0 | 2583.0 |
CH | 1850.0 | 2013.0 | 2037.0 | 2266.0 | 2306.0 | 2390.0 | 2471.0 | 2430.0 | 2566.0 | 2490.0 |
DE | 1595.0 | 1571.0 | 1655.0 | 1611.0 | 1823.0 | 1712.0 | 1742.0 | 2198.0 | 2448.0 | 2436.0 |
total_demand_dest = df_load_selected_com_minimal_dest.to_dict()
total_demand_origin = df_load_selected_com_minimal_origin.to_dict()
14. Build a Symmetric Linear Model#
from sympy.solvers import solve
from sympy import Symbol
lunl_minimal
['AT', 'CH', 'DE']
f12_s = Symbol("f12")
f13_s = Symbol("f13")
f23_s = Symbol("f23")
sols_s = {}
for year in years:
d1, d2, d3 = [total_demand_dest[year][i] for i in lunl_minimal]
eqns = [
f12_s * d2 + f13_s * d3 - 1,
f12_s * d1 + f23_s * d3 - 1,
f13_s * d1 + f23_s * d2 - 1
]
sols_s[year] = solve(eqns, f12_s, f13_s, f23_s, dict=True)[0]
print(sols_s)
{'2019': {f12: 0.000303742304713650, f13: 0.000274656260990437, f23: 0.000234855545200373}, '2018': {f12: 0.000302735191551942, f13: 0.000248627663530198, f23: 0.000247278747972678}, '2017': {f12: 0.000294397077859187, f13: 0.000241881058852468, f23: 0.000263404953970874}, '2016': {f12: 0.000289739080834145, f13: 0.000213191336331364, f23: 0.000244485315266896}, '2015': {f12: 0.000267589995791711, f13: 0.000210058952114270, f23: 0.000245727832518760}, '2014': {f12: 0.000276652003414130, f13: 0.000197898196168358, f23: 0.000244276189731357}, '2013': {f12: 0.000266398955631746, f13: 0.000196170023326037, f23: 0.000221861771056308}, '2012': {f12: 0.000228579971516024, f13: 0.000202252351781648, f23: 0.000237402502087569}, '2011': {f12: 0.000204213961033914, f13: 0.000194439124177686, f23: 0.000203531984370781}, '2010': {f12: 0.000205001189426696, f13: 0.000200963480430019, f23: 0.000193137080341069}}
Define the model
float(sols_s["2019"][f12_s])
0.00030374230471365
def gravity_model(coef_o_d, op, dq, all_coeff):
g = float(all_coeff[coef_o_d] * op * dq)
return g
total_demand_origin["2019"]["AT"]
1502.0
gravity_model(f12_s, total_demand_origin["2019"]["AT"], total_demand_dest["2019"]["CH"], sols_s["2019"])
844.0087421078192
gravity_model(f13_s, total_demand_origin["2019"]["AT"], total_demand_dest["2019"]["DE"], sols_s["2019"])
657.9912578921808
gravity_model(f23_s, total_demand_origin["2019"]["CH"], total_demand_dest["2019"]["DE"], sols_s["2019"])
74.91891891891892
The results are far from being correct
15. Introduce Symmetry Breaking#
lunl_minimal
['AT', 'CH', 'DE']
f12 = Symbol("f12")
f13 = Symbol("f13")
f21 = Symbol("f21")
f23 = Symbol("f23")
f31 = Symbol("f31")
f32 = Symbol("f32")
sols = {}
for year in years:
d1, d2, d3 = [total_demand_dest[year][i] for i in lunl_minimal]
eqns = [
f12 * d2 + f13 * d3 - 1,
f21 * d1 + f23 * d3 - 1,
f31 * d1 + f32 * d2 - 1
]
sols[year] = solve(eqns, f12, f13, f21, f23, f31, f32, dict=True)[0]
print(sols)
{'2019': {f31: 0.000485672656629432 - 0.898494414764449*f32, f21: 0.000485672656629432 - 0.774647887323944*f23, f12: 0.000540540540540541 - 0.862162162162162*f13}, '2018': {f31: 0.000495049504950495 - 0.996534653465347*f32, f21: 0.000495049504950495 - 0.777722772277228*f23, f12: 0.000496770988574267 - 0.780427223050174*f13}, '2017': {f31: 0.000521920668058455 - 1.06315240083507*f32, f21: 0.000521920668058455 - 0.863778705636743*f23, f12: 0.000490918016691213 - 0.812469317623957*f13}, '2016': {f31: 0.000478011472275335 - 1.08317399617591*f32, f21: 0.000478011472275335 - 0.770076481835564*f23, f12: 0.000441306266548985 - 0.710944395410415*f13}, '2015': {f31: 0.000484730974309258 - 1.11778962675715*f32, f21: 0.000484730974309258 - 0.883664566165778*f23, f12: 0.000433651344319167 - 0.790546400693842*f13}, '2014': {f31: 0.000475511174512601 - 1.13647170708512*f32, f21: 0.000475511174512601 - 0.814075130765573*f23, f12: 0.000418410041841004 - 0.716317991631799*f13}, '2013': {f31: 0.000434216239687364 - 1.07294832826748*f32, f21: 0.000434216239687364 - 0.756404689535389*f23, f12: 0.000404694455685957 - 0.704977741804937*f13}, '2012': {f31: 0.000478011472275335 - 1.16156787762906*f32, f21: 0.000478011472275335 - 1.05066921606119*f23, f12: 0.000411522633744856 - 0.904526748971193*f13}, '2011': {f31: 0.000407000407000407 - 1.04436304436304*f32, f21: 0.000407000407000407 - 0.996336996336996*f23, f12: 0.00038971161340608 - 0.954014029618083*f13}, '2010': {f31: 0.000387146728610143 - 0.963995354239257*f32, f21: 0.000387146728610143 - 0.943089430894309*f23, f12: 0.000401606425702811 - 0.978313253012048*f13}}
year_slice = 3
system_y = np.array([1, 1, 1] * len(years[:year_slice]))
system_A_nested = [[
[total_demand_origin[year]["CH"], total_demand_origin[year]["DE"], 0, 0, 0, 0],
[0, 0, total_demand_origin[year]["AT"], total_demand_origin[year]["DE"], 0, 0],
[0, 0, 0, 0, total_demand_origin[year]["AT"], total_demand_origin[year]["CH"]]
] for year in years[:year_slice]
]
system_A = np.array(
[item for sublist in system_A_nested for item in sublist]
)
system_A_T = np.transpose(system_A)
system_x = np.matmul(
np.matmul(
system_A_T,
np.linalg.pinv(
np.matmul(
system_A, system_A_T,
)
)
),
system_y
)
system_x
array([4.62077592e-04, 2.38748790e-04, 4.80157321e-05, 2.41902148e-04,
5.76484352e-04, 5.31941301e-04])
system_x[0] * total_demand_origin["2019"]["AT"] * total_demand_dest["2019"]["CH"]
1283.9750053370456
system_x[1] * total_demand_origin["2019"]["AT"] * total_demand_dest["2019"]["DE"]
571.968089786805
fig, ax = plt.subplots(figsize=(12, 10))
sns.heatmap(
df_lunl_minimal["2019"], annot=True, fmt="0.1f", linewidths=.5, ax=ax
)
<AxesSubplot:xlabel='destination', ylabel='origin'>
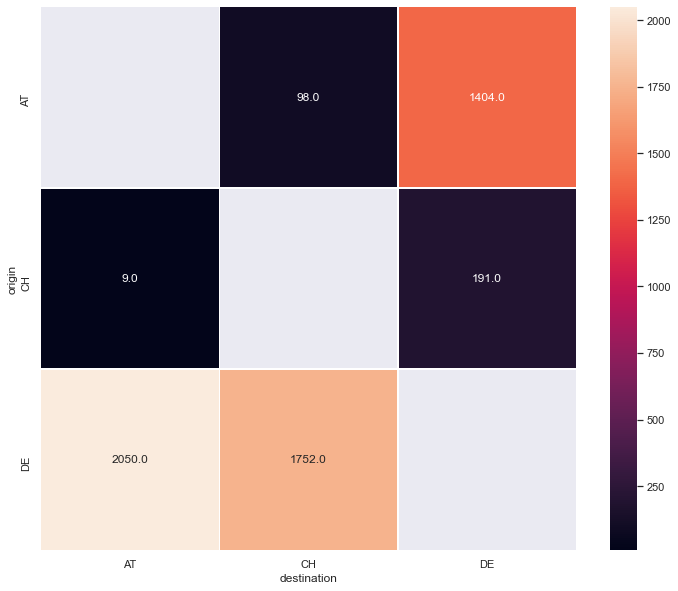
The results are still not as expected! We need to introduce more knowledge in to the coefficients. Now we need to introduce the gravity model!